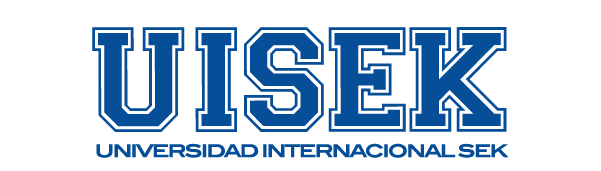
Por favor, use este identificador para citar o enlazar este ítem:
https://repositorio.uisek.edu.ec/handle/123456789/4864
Título : | Revisión sistemática y análisis de metodologías qué utilizan técnicas de minería de datos y aprendizaje automático para detección del trolling en las redes sociales |
Autor : | Carrión Jumbo, Joe Segovia Granda, Hugo Patricio |
Palabras clave : | DATA SCIENCE APRENDIZAJE DE MAQUINA ALGORITMOS TROLLING CIBERACOSO |
Fecha de publicación : | oct-2022 |
Editorial : | Universidad Internacional Sek |
Citación : | CT-MSIDS S454r/2022 |
Resumen : | El desarrollo de las redes sociales digitales ha facilitado a las personas mejorar su relacionamiento pues han permitido compartir por estos medios historias y experiencias expresadas en frases, imágenes, videos, audios, otros, toda esta información liberada dentro de grupos virtuales; sin embargo, no todo ha sido color de rosa ya que han existido también comportamientos anómalos en estos grupos y perfiles de usuarios que incitan a la violencia, el acoso, la burla, o la desinformación; a este tipo de comportamiento se lo denomina “trolling”. Las formas de expresar el trolling pueden ser variadas y van desde una muestra de irrespeto, sarcasmo, cyberbulling o influencia negativa hacia un grupo social o una persona, pudiendo ocasionar en algunos seres humanos problemas psicológicos complejos incluso suicidios en los casos más complicados. Para contrarrestar esta realidad muchos investigadores han desarrollado estudios que permiten identificar el trolling en las redes sociales mediante técnicas de minería de datos, software especializado o inteligencia artificial. El resultado de este trabajo permite a otros investigadores identificar técnicas, modelos, métodos y algoritmos utilizados para la detección del trolling. Es este trabajo se logró determinar los algoritmos usados más frecuentemente para este fin, entre estos se nombran a Random Forest, Regression Lineal, Support Vector Machine, Naïve Bayes, Kmeans, SMO, Gradient Boost, CNN. Algo que es preciso resaltar es la complejidad que tuvieron algunos investigadores a la hora de recabar datos de fuentes para su análisis, ya que la información no es libre, sin embargo, se logró identificar que la fuente de datos más usada pasa el análisis fue proveniente de la red social Twitter. También se logró concluir que existe un avance significativo en la identificación del trolling mediante técnicas de minería de datos y aprendizaje automático, no obstante se observa que ningún modelo entrega un alto porcentaje de certeza en la clasificación del contenido de tipo trolling y para la detección de perfiles de tipo troll, quizá la poca la poca información que entregan las empresas que son dueñas de los servicios de redes sociales sea la causa, así como las diferentes expresiones semánticas que tiene el lenguaje natural en diferentes culturas y países. |
Descripción : | The development of digital social networks has made it easier for people to improve their relationships as they have allowed them to share stories and experiences which are expressed in phrases, images, videos, audios, etc. All this information has been shared within virtual groups. However, not everything is all rosy, there are also abnormal behaviors in these groups and user profiles that incite violence, harassment, mockery or misinformation. This type of conduct is called “trolling”. Trolling can be expressed in many ways, such as, disrespect, sarcasm, cyberbullying or showing a negative influence on a group of people or an individual. This could cause psychological problems, which could even lead to suicide. To counter this reality, many researchers have developed studies that allow trolling to be identified through data mining techniques in social media, specialized software or artificial intelligence. The result of this work allows other researchers to identify techniques, models, methods and algorithms used for trolling detection. In this research, it was possible to determine the most frequently used algorithms for this purpose, among these are Random Forest, Linear Regression, Support Vector Machine, Naïve Bayes, Kmeans, SMO, Gradient Boost and CNN. Something that must be highlighted is the complexity that some researchers had when collecting data from sources for analysis, since the information is not free, however, it was possible to identify that the most used data source for the analysis was extracted from Twitter. It was also possible to conclude that there is a significant progress in the identification of trolling through data mining and machine learning techniques, however it is observed that no model provides a high percentage of certainty in the classification of trolling-type content and for the detection of trolling and troll-type profiles. It could be the limited information provided by the companies that own the social network services., as well as the different semantic expressions that natural language has in different cultures and countries. |
URI : | https://repositorio.uisek.edu.ec/handle/123456789/4864 |
Aparece en las colecciones: | Maestría en Sistemas de Información con mención en Data Science |
Ficheros en este ítem:
Fichero | Descripción | Tamaño | Formato | |
---|---|---|---|---|
Segovia Granda Hugo Patricio .pdf | 5,26 MB | Adobe PDF | Visualizar/Abrir |
Los ítems de DSpace están protegidos por copyright, con todos los derechos reservados, a menos que se indique lo contrario.