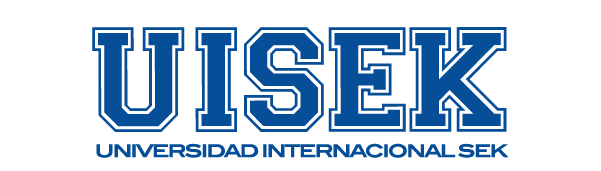
Por favor, use este identificador para citar o enlazar este ítem:
https://repositorio.uisek.edu.ec/handle/123456789/4256
Registro completo de metadatos
Campo DC | Valor | Lengua/Idioma |
---|---|---|
dc.contributor.advisor | Guevara Maldonado, César Byron | - |
dc.contributor.author | Freire López, Juan | - |
dc.date.accessioned | 2021-08-31T16:55:14Z | - |
dc.date.available | 2021-08-31T16:55:14Z | - |
dc.date.issued | 2021-08 | - |
dc.identifier.citation | CT-MSIDS F866m/2021 | es |
dc.identifier.uri | https://repositorio.uisek.edu.ec/handle/123456789/4256 | - |
dc.description | Esta investigación, se enfoca en Insotec, organización que se encuentra potenciando los procedimientos de sus áreas, incluyendo la evaluación crediticia que actualmente se realiza manualmente, este procedimiento analiza caso por caso y se aprueban las solicitudes dependiendo de la calificación, esto genera muchos inconvenientes como alta carga operativa y probabilidad de cometer errores en las evaluaciones. Para mejorar el proceso de evaluación crediticia de los clientes, el estudio propone crear un modelo basado en un algoritmo de inteligencia artificial que clasifique a los clientes de la organización como “buenos” o “malos” pagadores, en función de las diferentes variables consideradas importantes para el análisis. En la creación de modelo de clasificación se utiliza la base de datos de clientes de crédito de la organización con un histórico desde el año 2017 al 2020, este conjunto de datos cuenta con 18 variables y 63.896 registros. Adicionalmente, para la implementación del modelo de clasificación se utiliza la metodología CRISP-DM. Posteriormente, se prepara la información con el preprocesamiento de datos, en este paso se utiliza la técnica de eliminación de datos atípicos, con lo cual el conjunto de datos se reduce de 63.896 a 58.247. Finalmente, se seleccionan las variables con mayor importancia con el método de chi cuadrado, en este caso son 8 variables seleccionadas. El modelo es implementado con Random Forest, el cual arroja una precisión mayor al 97%, el porcentaje de error es del 2,8%, con el 2,1% falsos positivos y 11,1% falsos negativos para predecir. Finalmente, la creación del modelo ayuda a contar con una herramienta adicional que sirve para clasificar automáticamente a los clientes como “buenos” y “malos” pagadores, lo que puede ser utilizado para entregar créditos con más rapidez y con un menor grado de riesgo. Sin embargo, este modelo necesita ser desplegado como lo indica la metodología CRISP-DM, para que el conocimiento obtenido sea aprovechado por el cliente. | es |
dc.description.abstract | This research focuses on Insotec, a medium-sized entity that dedicates to granting microcredits. The organization is looking forward to enhancing different areas procedures, including the credit evaluation, which is carried out manually. Currently, analysts classify risk on a case-by-case basis, and applications are approved depending on the credit rating. This process generates many drawbacks, such as high operational load and the probability of making wrong evaluations. This study proposes to enhance the risk evaluation process by creating a model based on an artificial intelligence algorithm that classifies the organization's customers as good or bad payers based on the different variables considered essential for the analysis. Furthermore, the study uses the organization's credit customer database with a historic from 2017 to 2020. This dissertation proposes creating an algorithmic model using the CRISP-DM methodology to classify the entity's clients. First, the business and the data set are understood. Second, the information is prepared within the data preprocessing phase. This step applies the outlier elimination technique. In this phase, the dataset reduces from 63,896 to 58,247 records. Afterward, the chi-square technique selects the variables with the most significant importance method, which sets eight variables for the analysis. When the dataset is ready, the CRISP-DM methodology suggests selecting the techniques most suitable for the model. In this case, the Random Forest algorithm obtains the best precision results compared against other algorithms such as neural networks and decision trees. The Random Forest model throws a precision of 97%, an error of 2.8%, and a rate of 2.1% false positives and 11.1% false negatives to predict. Additionally, the analysis implements ten models with the cross-validation method for the model evaluation phase. Finally, creating the model leads to having an additional tool, which automates the clients' classification process as good and bad payers. This automation can be used to deliver loans faster and with less risk. However, the deployment phase needs to be executed as mentions de CRISP-DM methodology states, so that the client can make the most of the knowledge obtained by the model. | es |
dc.description.sponsorship | Uisek | es |
dc.language.iso | spa | es |
dc.publisher | Universidad Internacional Sek | es |
dc.rights | openAccess | es |
dc.subject | DATA SCIENCE | es |
dc.subject | RANDOM FOREST | es |
dc.subject | ARBOLES DE DECISIÓN | es |
dc.subject | CHI CUADRADO | es |
dc.subject | CLASIFICACIÓN DE CLIENTES DE CRÉDITO | es |
dc.title | Modelo de clasificación de riesgo crediticio utilizando Random Forest en financiera del Ecuador | es |
dc.type | info:eu-repo/semantics/masterThesis | es |
Aparece en las colecciones: | Maestría en Sistemas de Información con mención en Data Science |
Ficheros en este ítem:
Fichero | Descripción | Tamaño | Formato | |
---|---|---|---|---|
Freire López, Juan.pdf | 1,5 MB | Adobe PDF | Visualizar/Abrir |
Los ítems de DSpace están protegidos por copyright, con todos los derechos reservados, a menos que se indique lo contrario.